检查您的理解情况:卷积
将一个二维 3x3 卷积过滤器应用到一个二维 4x4 输入特征图(未添加内边距):
输出特征图是形状什么的?
2x2
当 3x3 过滤器在 4x4 特征图上滑动时,过滤器可以放置在 4 个不同位置,生成一个 2x2 输出特征图:
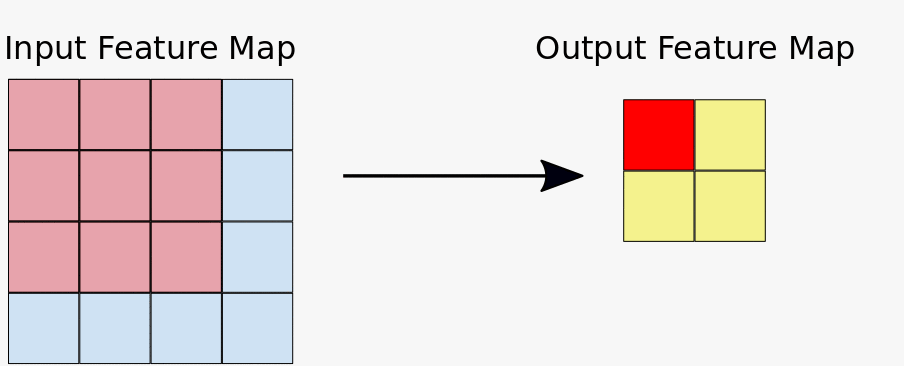
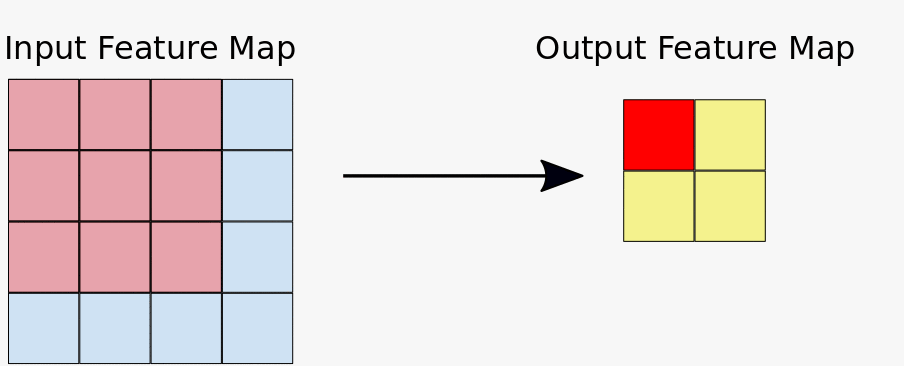
3x3
虽然滤波器本身为 3x3,但输出特征图可能较小,这是因为在 4x4 输入特征图上可放置滤波器的位置不到 9(3 乘以 3)个位置。
4x4
为了生成大小与输入特征图无内边距的输出特征图,卷积过滤器的形状必须为 1x1。大于 1x1 的过滤器将生成小于输入特征图的输出特征图。由于我们的过滤器为 3x3,因此输出特征图必须小于 4x4。