瞭解你的理解:卷積
雙維度 3x3 卷積篩選器套用至二維 4x4 輸入特徵對應 (未新增邊框間距):
輸出特徵圖的形狀為何?
2x2
3x3 篩選器會在 4x4 功能對應上滑動,因此可以放置 4 個不重複的位置,導致產生 2x2 輸出的特徵地圖: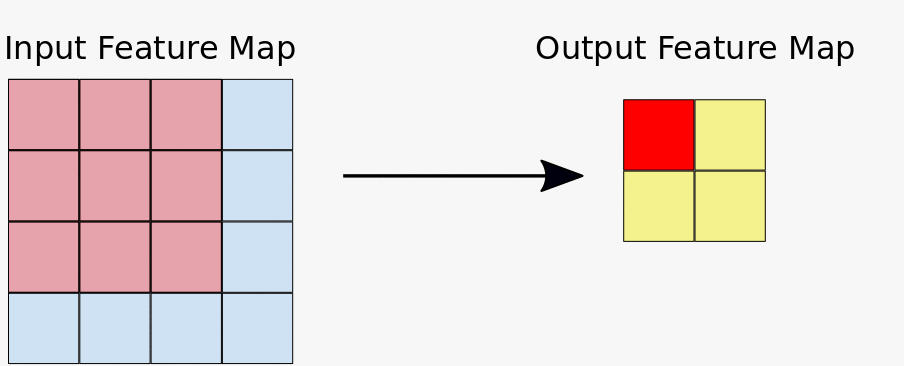
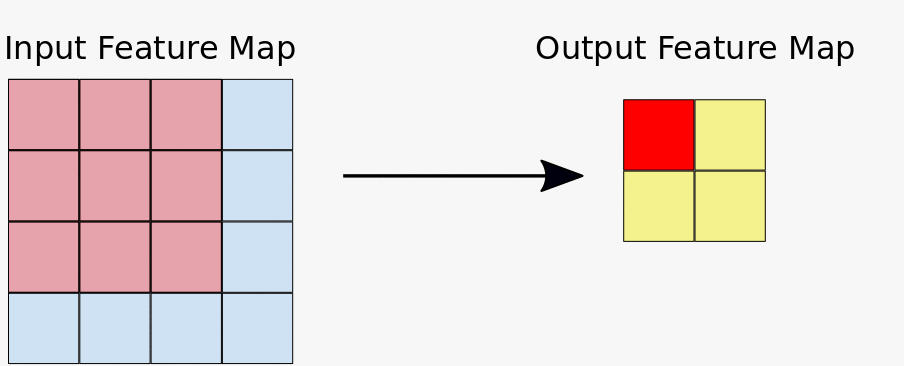
三對三籃球
雖然篩選器本身是 3x3,但輸出功能對應關係較少,因為在 4x4 輸入功能地圖上可放置篩選器的可能位置少於 9 (3 倍 3) 。
四輪驅動
如要產生與邊框間距維度相同的輸出特徵對應,且沒有邊框間距,卷積篩選器的形狀必須是 1x1。大於 1x1 的篩選器會產生輸出特徵對應,小於輸入特徵對應。由於我們的篩選條件是 3x3,因此輸出特徵對應必須小於 4x4。