इस पेज में बताया गया है कि किसी मॉडल को फ़ेडरेटेड औसत लर्निंग प्रोसेस और तय गॉसियन नॉइज़ के साथ ट्रेनिंग देने के लिए, उपयोगकर्ता को डिवाइस पर मनमुताबिक बनाने की सुविधा से मिले फ़ेडरेटेड लर्निंग एपीआई का इस्तेमाल कैसे किया जा सकता है.
शुरू करने से पहले
शुरू करने से पहले, अपने टेस्ट डिवाइस पर यह तरीका अपनाएं:
पक्का करें कि OnDevicePersonalization मॉड्यूल इंस्टॉल हो. यह मॉड्यूल, अप्रैल 2024 में अपने-आप अपडेट होने के लिए उपलब्ध हो गया था.
# List the modules installed on the device adb shell pm list packages --apex-only --show-versioncode
पक्का करें कि नीचे दिया गया मॉड्यूल 341717000 या इसके बाद के वर्शन कोड के साथ सूची में मौजूद है:
package:com.google.android.ondevicepersonalization versionCode:341717000
अगर वह मॉड्यूल सूची में नहीं है, तो सेटिंग > सुरक्षा और निजता > अपडेट > Google Play का सिस्टम अपडेट पर जाकर यह पक्का करें कि आपका डिवाइस अप-टू-डेट है. ज़रूरत के हिसाब से अपडेट करें चुनें.
फ़ेडरेटेड लर्निंग से जुड़ी सभी नई सुविधाएं चालू करें.
# Enable On-Device Personalization apk. adb shell device_config put on_device_personalization global_kill_switch false # Enable On-Device Personalization APIs. adb shell device_config put on_device_personalization enable_ondevicepersonalization_apis true # Enable On-Device Personalization overriding. adb shell device_config put on_device_personalization enable_personalization_status_override true adb shell device_config put on_device_personalization personalization_status_override_value true # Enable Federated Compute apk. adb shell device_config put on_device_personalization federated_compute_kill_switch false
फ़ेडरेटेड लर्निंग टास्क बनाएं
डायग्राम में दिए गए नंबरों के बारे में ज़्यादा जानकारी, नीचे दिए गए आठ चरणों में दी गई है.
फ़ेडरेटेड कंप्यूट सर्वर को कॉन्फ़िगर करना
फ़ेडरेटेड लर्निंग, एक मैप-रिड्यूस है. यह फ़ेडरेटेड कंप्यूट सर्वर (रिड्यूसर) और क्लाइंट (मैपर) के सेट पर चलता है. फ़ेडरेटेड कंप्यूट सर्वर, फ़ेडरेटेड लर्निंग के हर टास्क के चल रहे मेटाडेटा और मॉडल की जानकारी को मैनेज करता है. बड़े लेवल पर:
- फ़ेडरेटेड लर्निंग डेवलपर, नया टास्क बनाता है और टास्क के रनिंग मेटाडेटा और मॉडल की जानकारी, दोनों को सर्वर पर अपलोड करता है.
- जब कोई फ़ेडरेटेड कंप्यूट क्लाइंट, सर्वर को टास्क असाइन करने का नया अनुरोध करता है, तो सर्वर टास्क की ज़रूरी शर्तों की जांच करता है और ज़रूरी शर्तें पूरी करने वाले टास्क की जानकारी दिखाता है.
- एक बार फ़ेडरेटेड कंप्यूट क्लाइंट लोकल कंप्यूटेशन पूरा कर लेता है, तो यह कंप्यूटेशन के इन नतीजों को सर्वर को भेजता है. इसके बाद, सर्वर इन कंप्यूटेशन के नतीजों को एग्रीगेशन और नॉइज़ करता है और नतीजों को आखिरी मॉडल पर लागू करता है.
इन कॉन्सेप्ट के बारे में ज़्यादा जानने के लिए, ये लेख पढ़ें:
- फ़ेडरेटेड लर्निंग: सेंट्रलाइज़्ड ट्रेनिंग डेटा के बिना कोलैबोरेटिव मशीन लर्निंग
- बड़े पैमाने पर फ़ेडरेटेड लर्निंग की ओर: सिस्टम डिज़ाइन (SysML 2019)
ओडीपी, फ़ेडरेटेड लर्निंग के बेहतर वर्शन का इस्तेमाल करता है. इसमें मॉडल पर लागू करने से पहले, एग्रीगेट पर कैलिब्रेट किया गया (केंद्रीकृत) शोर लागू किया जाता है. नॉइज़ स्केल से यह पक्का होता है कि एग्रीगेट, डिफ़रेंशियल प्राइवसी को बनाए रखता है.
पहला चरण. फ़ेडरेटेड कंप्यूट सर्वर बनाना
अपना खुद का फ़ेडरल कंप्यूट सर्वर सेट अप करने के लिए, फ़ेडरल कंप्यूट प्रोजेक्ट में दिए गए निर्देशों का पालन करें.
दूसरा चरण. सेव किया गया फ़ंक्शनल मॉडल तैयार करना
सेव की गई 'FunctionalModel' फ़ाइल तैयार करें. किसी 'मॉडल' को 'FunctionalModel' में बदलने के लिए, 'functional_model_from_keras' का इस्तेमाल करें और इस 'FunctionalModel' को 'SavedModel' के तौर पर क्रम में लगाने के लिए 'save_Functional_model' का इस्तेमाल करें.
functional_model = tff.learning.models.functional_model_from_keras(keras_model=model)
tff.learning.models.save_functional_model(functional_model, saved_model_path)
तीसरा चरण. फ़ेडरेटेड कंप्यूट सर्वर का कॉन्फ़िगरेशन बनाना
एक fcp_server_config.json
तैयार करें, जिसमें नीतियां, फ़ेडरेटेड लर्निंग सेटअप, और अलग-अलग निजता सेटिंग शामिल हों. उदाहरण:
# Identifies the set of client devices that will participate.
population_name: "my_new_population"
# Options you can choose:
# * TRAINING_ONLY: Only one training task will be generated under this
# population.
# * TRAINING_AND_EVAL: One training task and one evaluation task will be
# generated under this population.
# * EVAL_ONLY: Only one evaluation task will be generated under this
# population.
mode: TRAINING_AND_EVAL
policies {
# Policy for sampling on-device examples. It is checked every time a
# device attempts to start a new training.
min_separation_policy {
# The minimum number of rounds before the same client participated.
minimum_separation: 3
}
# Policy for releasing training results to developers. It is checked
# when uploading a new task to the Federated Compute Server.
model_release_policy {
# Server stops training when number of training rounds reaches this
# number.
num_max_training_rounds: 1000
}
}
# Federated learning setups. They are applied inside Task Builder.
federated_learning {
learning_process {
# Use FED_AVG to build federated learning process. Options you can
# choose:
# * FED_AVG: Federated Averaging algorithm
# (https://arxiv.org/abs/2003.00295)
# * FED_SDG: Federated SGD algorithm
# (https://arxiv.org/abs/1602.05629)
type: FED_AVG
# Optimizer used at client side training. Options you can choose:
# * ADAM
# * SGD
client_optimizer: SGD
# Learning rate used at client side training.
client_learning_rate: 0.01
# Optimizer used at server side training. Options you can choose:
# * ADAM
# * SGD
server_optimizer: ADAM
# Learning rate used at server side training.
sever_learning_rate: 1
runtime_config {
# Number of participating devices for each round of training.
report_goal: 2000
}
# List of metrics to be evaluated by the model during training and
# evaluation. Federated Compute Server provides a list of allowed
# metrics.
metrics {
name: "auc-roc"
}
metrics {
name: "binary_accuracy"
}
}
# Whether or not to generate a corresponding evaluation task under the same
# population. If this field isn't set, only one training task is
# generated under this population.
evaluation {
# The task id under the same population of the source training task that
# this evaluation task evaluates.
source_training_task_id: 1
# Decides how checkpoints from the training task are chosen for
# evaluation.
# * every_k_round: the evaluation task randomly picks one checkpoint
# from the past k rounds of training task checkpoints.
# * every_k_hour: the evaluation task randomly picks one checkpoint
# from the past k hours of training task checkpoints.
checkpoint_selector: "every_1_round"
# The traffic of this evaluation task in this population.
evaluation_traffic: 0.1
# Number of participating devices for each round of evaluation.
report_goal: 200
}
}
# Differential Privacy setups. They are applied inside the Task Builder.
differential_privacy {
# The DP aggregation algorithm you want to use. Options you can choose:
# * FIXED_GAUSSIAN: Federated Learning DP-SGD with fixed clipping norm
# described in "Learning Differentially Private Recurrent
# Language Models" (https://arxiv.org/abs/1710.06963).
# * ADAPTIVE_GAUSSIAN: Federated Learning DP-SGD with quantile-based clip
# norm estimation described in "Differentially Private
# Learning with Adaptive Clipping"
# (https://arxiv.org/abs/1905.03871).
# * TREE: DP-FTRL algorithm described in "Practical and Private (Deep)
# Learning without Sampling or Shuffling"
# (https://arxiv.org/abs/2103.00039).
# * ADADPTIVE_TREE: DP-FTRL with adaptive clipping norm descirbed in
# "Differentially Private Learning with Adaptive Clipping"
# (https://arxiv.org/abs/1905.03871).
type: FIXED_GAUSSIAN
# Noise multiplier for the Gaussian noise.
noise_multiplier: 0.1
# The value of the clipping norm.
clip_norm: 0.1
}
चरण 4. संघीय गणना सर्वर पर ज़िप कॉन्फ़िगरेशन सबमिट करें.
ज़िप फ़ाइल और fcp_server_config.json
को फ़ेडरेटेड कंप्यूट सर्वर पर सबमिट करें.
task_builder_client --task_builder_server='http://{federated_compute_server_endpoint}' --saved_model='saved_model' --task_config='fcp_server_config.json'
फ़ेडरेटेड कंप्यूट सर्वर एंडपॉइंट वह सर्वर है जिसे आपने पहले चरण में सेट अप किया था.
LiteRT बिल्ट-इन ऑपरेटर लाइब्रेरी, सिर्फ़ कुछ ही TensorFlow ऑपरेटर (TensorFlow ऑपरेटर चुनें) के साथ काम करती है. OnDevicePersonalization मॉड्यूल के अलग-अलग वर्शन पर काम करने वाले ऑपरेटर का सेट अलग-अलग हो सकता है. यह पक्का करने के लिए कि टास्क काम करेगा, टास्क बनाने के दौरान टास्क बिल्डर में ऑपरेटर की पुष्टि की प्रक्रिया की जाती है.
टास्क के मेटाडेटा में, OnDevicePersonalization मॉड्यूल का कम से कम काम करने वाला वर्शन शामिल किया जाएगा. यह जानकारी, टास्क बिल्डर के जानकारी वाले मैसेज में देखी जा सकती है.
I1023 22:16:53.058027 139653371516736 task_builder_client.py:109] Success! Tasks are built, and artifacts are uploaded to the cloud. I1023 22:16:53.058399 139653371516736 task_builder_client.py:112] applied_algorithms { learning_algo: FED_AVG client_optimizer: SGD server_optimizer: SGD dp_aggregator: FIXED_GAUSSIAN } metric_results { accepted_metrics: "binary_accuracy, binary_crossentropy, recall, precision, auc-roc, auc-pr" } dp_hyperparameters { dp_delta: 0.000001 dp_epsilon: 6.4 noise_multiplier: 1.0 dp_clip_norm: 1.0 num_training_rounds: 10000 } I1023 22:16:53.058594 139653371516736 task_builder_client.py:113] training_task { min_client_version: "341912000" } eval_task { min_client_version: "341812000" }
फ़ेडरेटेड कंप्यूट सर्वर इस टास्क को उन सभी डिवाइसों को असाइन करेगा जिन पर OnDevicePersonalization मॉड्यूल वाले ऐप्लिकेशन का इस्तेमाल होता है. इन डिवाइसों का वर्शन 341812000 से बाद का है.
अगर आपके मॉडल में ऐसी कार्रवाइयां शामिल हैं जो OnDevicePersonalization के किसी भी मॉड्यूल के साथ काम नहीं करती हैं, तो टास्क बनाने के दौरान गड़बड़ी का मैसेज जनरेट होगा.
common.TaskBuilderException: Cannot build the ClientOnlyPlan: Please contact Google to register these ops: {'L2Loss': 'L2LossOp<CPUDevice, float>'} . Stop building remaining artifacts.
GitHub में, इस्तेमाल किए जा सकने वाले फ़्लेक्स ऑपरेटर की पूरी सूची देखी जा सकती है.
Android फ़ेडरेटेड कंप्यूट APK बनाएं
Android फ़ेडरेटेड कंप्यूट APK बनाने के लिए, आपको अपने AndroidManifest.xml
में फ़ेडरल कंप्यूट सर्वर यूआरएल एंडपॉइंट तय करना होगा, जिससे आपका फ़ेडरेटेड कंप्यूट क्लाइंट कनेक्ट होता है.
पांचवां चरण. फ़ेडरेटेड कंप्यूट सर्वर यूआरएल एंडपॉइंट के बारे में बताएं
अपने AndroidManifest.xml
में, फ़ेडरेटेड कंप्यूट सर्वर का यूआरएल एंडपॉइंट डालें. यह वह एंडपॉइंट है जिसे आपने पहले चरण में सेट अप किया था. इससे आपका फ़ेडरेटेड कंप्यूट क्लाइंट कनेक्ट होता है.
<!-- Contents of AndroidManifest.xml -->
<manifest xmlns:android="http://schemas.android.com/apk/res/android"
package="com.example.odpsample" >
<application android:label="OdpSample">
<!-- XML resource that contains other ODP settings. -->
<property android:name="android.ondevicepersonalization.ON_DEVICE_PERSONALIZATION_CONFIG"
android:resource="@xml/OdpSettings"></property>
<!-- The service that ODP will bind to. -->
<service android:name="com.example.odpsample.SampleService"
android:exported="true" android:isolatedProcess="true" />
</application>
</manifest>
<property>
टैग में बताई गई एक्सएमएल रिसॉर्स फ़ाइल में, <service>
टैग में सेवा क्लास की जानकारी भी होनी चाहिए. साथ ही, उस फ़ेडरेटेड कंप्यूट सर्वर के यूआरएल एंडपॉइंट की जानकारी भी होनी चाहिए जिससे फ़ेडरेटेड कंप्यूट क्लाइंट कनेक्ट होगा:
<!-- Contents of res/xml/OdpSettings.xml -->
<on-device-personalization>
<!-- Name of the service subclass -->
<service name="com.example.odpsample.SampleService">
<!-- If you want to use federated compute feature to train a model,
specify this tag. -->
<federated-compute-settings url="https://fcpserver.com/" />
</service>
</on-device-personalization>
छठा चरण. IsolatedWorker#onTrainingExample
एपीआई को लागू करें
ट्रेनिंग का डेटा जनरेट करने के लिए, डिवाइस पर उपयोगकर्ता के मनमुताबिक अनुभव पाने की सुविधा से जुड़ा सार्वजनिक एपीआई IsolatedWorker#onTrainingExample
लागू करें.
IsolatedProcess
में चल रहे कोड के पास, डिवाइस पर चल रहे नेटवर्क, लोकल डिस्क या अन्य सेवाओं का सीधा ऐक्सेस नहीं होता. हालांकि, ये एपीआई उपलब्ध हैं:
- 'getRemoteData' - डेवलपर के ऑपरेट किए जा रहे रिमोट बैकएंड से डाउनलोड किया गया, बदलाव न किया जा सकने वाला की-वैल्यू डेटा. हालांकि, यह ज़रूरी नहीं है कि यह हमेशा उपलब्ध हो.
- 'getLocalData' - डेवलपर के पास, डिवाइस में सेव किए गए बदलाव किए जा सकने वाले की-वैल्यू डेटा को ऐक्सेस करने का विकल्प होता है. हालांकि, ऐसा सिर्फ़ तब किया जा सकता है, जब डेवलपर ने ऐसा किया हो.
- 'UserData' - प्लैटफ़ॉर्म से मिला उपयोगकर्ता का डेटा.
- 'getLogReader' - यह REQUESTS और EVENTS टेबल के लिए DAO दिखाता है.
उदाहरण:
@Override public void onTrainingExample(
@NonNull TrainingExampleInput input,
@NonNull Consumer<TrainingExampleOutput> consumer) {
// Check if the incoming training task is the task we want.
if (input.getPopulationName() == "my_new_population") {
TrainingExampleOutput result = new TrainingExampleOutput.Builder():
RequestLogRecord record = this.getLogReader().getRequestLogRecord(1);
int count = 1;
// Iterate logging event table.
for (ContentValues contentValues: record.rows()) {
Features features = Features.newBuilder()
// Retrieve carrier from user info.
.putFeature("carrier", buildFeature(mUserData.getCarrier()))
// Retrieve features from logging info.
.putFeature("int_feature_1",
buildFeature(contentValues.get("int_feature_1")
result.addTrainingExample(
Example.newBuilder()
.setFeatures(features).build().toByteArray())
.addResumptionToken(
String.format("token%d", count).getBytes()))
.build();
count++;
}
consumer.accept(result.build());
}
}
सातवां चरण. बार-बार होने वाला ट्रेनिंग टास्क शेड्यूल करें.
डिवाइस पर उपयोगकर्ताओं के हिसाब से कॉन्टेंट दिखाने की सुविधा, डेवलपर को FederatedComputeScheduler
उपलब्ध कराती है. इसकी मदद से, वे फ़ेडरेटेड कंप्यूट जॉब को शेड्यूल या रद्द कर सकते हैं. IsolatedWorker
की मदद से इसे कॉल करने के लिए, शेड्यूल करने या असाइन किए गए डाउनलोड के पूरा होने पर, अलग-अलग विकल्प उपलब्ध हैं. दोनों के उदाहरण यहां दिए गए हैं.
शेड्यूल पर आधारित विकल्प.
IsolatedWorker#onExecute
मेंFederatedComputeScheduler#schedule
को कॉल करें.@Override public void onExecute( @NonNull ExecuteInput input, @NonNull Consumer<ExecuteOutput> consumer ) { if (input != null && input.getAppParams() != null && input.getAppParams().getString("schedule_training") != null) { if (input.getAppParams().getString("schedule_training").isEmpty()) { consumer.accept(null); return; } TrainingInterval interval = new TrainingInterval.Builder() .setMinimumInterval(Duration.ofSeconds(10)) .setSchedulingMode(2) .build(); FederatedComputeScheduler.Params params = new FederatedComputeScheduler .Params(interval); FederatedComputeInput fcInput = new FederatedComputeInput.Builder() .setPopulationName( input.getAppParams().getString("schedule_training")).build(); mFCScheduler.schedule(params, fcInput); ExecuteOutput result = new ExecuteOutput.Builder().build(); consumer.accept(result); } }
डाउनलोड पूरा होने का विकल्प. अगर ट्रेनिंग टास्क को शेड्यूल करने के लिए, किसी ऐसे डेटा या प्रोसेस पर निर्भर करना है जो एक साथ काम नहीं करती हैं, तो
IsolatedWorker#onDownloadCompleted
मेंFederatedComputeScheduler#schedule
को कॉल करें.
पुष्टि
यहां बताया गया है कि फ़ेडरेटेड लर्निंग टास्क ठीक से चल रहा है या नहीं, इसकी पुष्टि कैसे की जा सकती है.
चरण 8. पुष्टि करें कि फ़ेडरेटेड लर्निंग टास्क सही तरीके से चल रहा है या नहीं.
सर्वर साइड एग्रीगेशन के हर राउंड में, एक नया मॉडल चेकपॉइंट और एक नई मेट्रिक फ़ाइल जनरेट होती है.
ये मेट्रिक, की-वैल्यू पेयर की JSON फ़ॉर्मैट वाली फ़ाइल में होती हैं. यह फ़ाइल, तीसरे चरण में तय की गई Metrics
की सूची से जनरेट होगी. मेट्रिक की JSON फ़ाइल का उदाहरण यहां दिया गया है:
{"server/client_work/train/binary_accuracy":0.5384615659713745, "server/client_work/train/binary_crossentropy":0.694046676158905, "server/client_work/train/recall":0.20000000298023224, "server/client_work/train/precision":0.3333333432674408, "server/client_work/train/auc-roc":0.3500000238418579, "server/client_work/train/auc-pr":0.44386863708496094, "server/finalizer/update_non_finite":0.0}
मॉडल मेट्रिक पाने और ट्रेनिंग की परफ़ॉर्मेंस को मॉनिटर करने के लिए, नीचे दी गई स्क्रिप्ट से मिलती-जुलती स्क्रिप्ट का इस्तेमाल किया जा सकता है:
import collections
import json
import matplotlib.pyplot as plt
from google.cloud import storage
# The population_name you set in fcp_server_config.json in Step 3.
POPULATION_NAME = 'my_new_population'
# The Google Cloud storage you set in Step 1.
GCS_BUCKET_NAME = 'fcp-gcs'
NUM_TRAINING_ROUND = 1000
storage_client = storage.Client()
bucket = storage_client.bucket(GCS_BUCKET_NAME)
metrics = collections.defaultdict(list)
for i in range(NUM_TRAINING_ROUND):
blob = bucket.blob('{}/{}/1/{}/s/0/metrics'.format(GCS_BUCKET_NAME, POPULATION_NAME, i+1))
with blob.open("r") as f:
metric = json.loads(f.read())
for metric_name in metric.keys():
metrics[metric_name].append(metric[metric_name])
for metric_name in metrics:
print(metric_name)
plt.plot(metrics[metric_name])
plt.show()
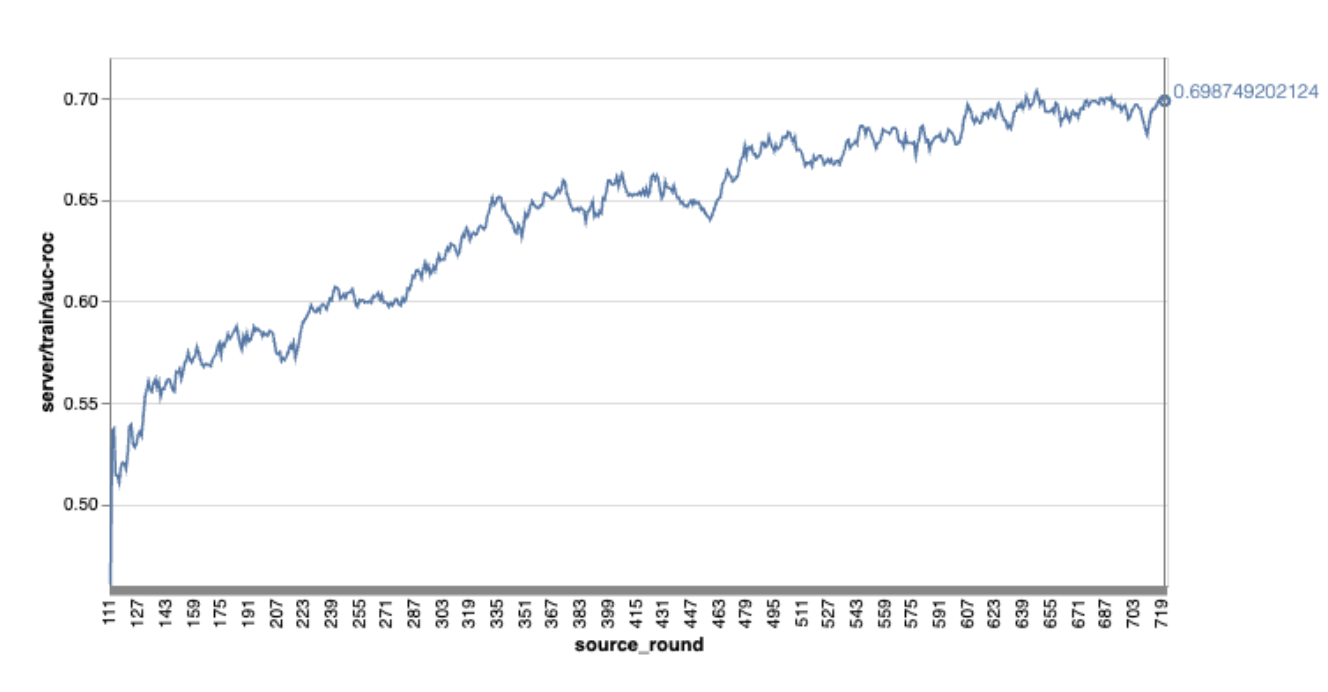
ध्यान दें कि पिछले उदाहरण ग्राफ़ में:
- एक्स-ऐक्सिस, राउंड ट्रेनिंग की संख्या है.
- y-ऐक्सिस, हर राउंड के auc-roc की वैल्यू है.
उपयोगकर्ता के डिवाइस पर पर्सनलाइज़ेशन के लिए, इमेज क्लासिफ़िकेशन मॉडल को ट्रेनिंग देना
इस ट्यूटोरियल में, ईएमएनआईएसटी डेटासेट का इस्तेमाल यह दिखाने के लिए किया गया है कि ओडीपी पर फ़ेडरेटेड लर्निंग टास्क कैसे चलाया जाता है.
पहला चरण. tff.learning.models.FunctionalModel बनाएं
def get_image_classification_input_spec():
return (
tf.TensorSpec([None, 28, 28, 1], tf.float32),
tf.TensorSpec([None, 1], tf.int64),
)
def create_and_save_image_classification_functional_model(
model_path: str,
) -> None:
keras_model = emnist_models.create_original_fedavg_cnn_model(
only_digits=True
)
functional_model = tff.learning.models.functional_model_from_keras(
keras_model=keras_model,
input_spec=get_image_classification_input_spec(),
loss_fn=tf.keras.losses.SparseCategoricalCrossentropy(),
)
tff.learning.models.save_functional_model(functional_model, model_path)
- emnist_models में, emnist keras मॉडल की जानकारी देखी जा सकती है.
- फ़िलहाल, TfLite में tf.sparse.SparseTensor या tf.RaggedTensor के लिए अच्छी सुविधा उपलब्ध नहीं है. मॉडल बनाते समय, tf.Tensor का ज़्यादा से ज़्यादा इस्तेमाल करने की कोशिश करें.
- लर्निंग प्रोसेस बनाते समय, ओडीपी टास्क बिल्डर, सभी मेट्रिक को ओवरराइट कर देगा. इसके लिए, कोई मेट्रिक तय करने की ज़रूरत नहीं है. इस विषय के बारे में ज़्यादा जानकारी दूसरे चरण में दी गई है. टास्क बिल्डर कॉन्फ़िगरेशन बनाएं.
दो तरह के मॉडल इनपुट काम करते हैं:
टाइप 1. एक tuple(features_tensor, Label_tensor).
- मॉडल बनाते समय, input_spec इस तरह दिखता है:
def get_input_spec(): return ( tf.TensorSpec([None, 28, 28, 1], tf.float32), tf.TensorSpec([None, 1], tf.int64), )
- डिवाइस पर ट्रेनिंग डेटा जनरेट करने के लिए, ऊपर दिए गए कोड को ODP के सार्वजनिक API IsolatedWorker#onTrainingExamples के इस लागू किए गए कोड के साथ जोड़ें:
return tf.train.Example( features=tf.train.Features( feature={ 'x': tf.train.Feature( float_list=tf.train.FloatList(value=[1.0] * 784) ), 'y': tf.train.Feature( int64_list=tf.train.Int64List( value=[1] ) ), } ) ).SerializeToString()
टाइप 2.
Tuple(Dict[feature_name, feature_tensor], label_tensor)
- मॉडल बनाते समय, input_spec इस तरह दिखता है:
def get_input_spec() -> ( Tuple[collections.OrderedDict[str, tf.TensorSpec], tf.TensorSpec] ): return ( collections.OrderedDict( [('feature-1', tf.TensorSpec([None, 1], tf.float32)), ('feature-2', tf.TensorSpec([None, 1], tf.float32))] ), tf.TensorSpec([None, 1], tf.int64), )
- ट्रेनिंग डेटा जनरेट करने के लिए, पिछले कोड को ओडीपी पब्लिक एपीआई IsolatedWorker#onTrainingExamples को लागू करने की इस प्रोसेस से जोड़ें:
return tf.train.Example( features=tf.train.Features( feature={ 'feature-1': tf.train.Feature( float_list=tf.train.FloatList(value=[1.0]) ), 'feature-2': tf.train.Feature( float_list=tf.train.FloatList(value=[2.0]) ), 'my_label': tf.train.Feature( int64_list=tf.train.Int64List( value=[1] ) ), } ) ).SerializeToString()
- टास्क बिल्डर के कॉन्फ़िगरेशन में, label_name को रजिस्टर करना न भूलें.
mode: TRAINING_AND_EVAL # Task execution mode population_name: "my_example_model" label_name: "my_label"
लर्निंग प्रोसेस बनाते समय, ओडीपी डीपी को अपने-आप मैनेज करता है. इसलिए, फ़ंक्शनल मॉडल बनाते समय कोई ग़ैर-ज़रूरी जानकारी जोड़ने की ज़रूरत नहीं है.
सेव किए गए इस फ़ंक्शनल मॉडल का आउटपुट, GitHub रिपॉज़िटरी में मौजूद सैंपल की तरह दिखना चाहिए.
दूसरा चरण. टास्क बिल्डर कॉन्फ़िगरेशन बनाना
आपको GitHub रिपॉज़िटरी में टास्क बिल्डर कॉन्फ़िगरेशन के सैंपल मिल सकते हैं.
ट्रेनिंग और आकलन वाली मेट्रिक
मेट्रिक से उपयोगकर्ता का डेटा लीक हो सकता है. इसलिए, टास्क बिल्डर में उन मेट्रिक की सूची होगी जिन्हें लर्निंग प्रोसेस जनरेट और रिलीज़ कर सकती है. हमारे GitHub डेटा स्टोर में, पूरी सूची देखी जा सकती है.
नया टास्क बिल्डर कॉन्फ़िगरेशन बनाते समय, मेट्रिक की सूची का सैंपल यहां दिया गया है:
federated_learning { learning_process { metrics { name: "binary_accuracy" } metrics { name: "binary_crossentropy" } metrics { name: "recall" } metrics { name: "precision" } metrics { name: "auc-roc" } metrics { name: "auc-pr" } } }
अगर आपकी पसंद की मेट्रिक मौजूदा सूची में नहीं हैं, तो हमसे संपर्क करें.
डीपी कॉन्फ़िगरेशन
डीपी से जुड़े कुछ कॉन्फ़िगरेशन के लिए यह जानकारी देना ज़रूरी है:
policies { min_separation_policy { minimum_separation: 1 } model_release_policy { num_max_training_rounds: 1000 dp_target_epsilon: 10 dp_delta: 0.000001 } } differential_privacy { type: FIXED_GAUSSIAN clip_norm: 0.1 noise_multiplier: 0.1 }
- पुष्टि करने के लिए,
dp_target_epsilon
याnoise_mulitipiler
मौजूद है: (noise_to_epsilon
epislon_to_noise
). - आपको GitHub रिपॉज़िटरी में ये डिफ़ॉल्ट सेटिंग मिल सकती हैं.
- पुष्टि करने के लिए,
तीसरा चरण. सेव किए गए मॉडल और टास्क बिल्डर कॉन्फ़िगरेशन को किसी भी डेवलपर के क्लाउड स्टोरेज पर अपलोड करें
टास्क बिल्डर कॉन्फ़िगरेशन अपलोड करते समय, artifact_building फ़ील्ड अपडेट करना न भूलें.
चरण 4. (ज़रूरी नहीं) नया टास्क बनाए बिना आर्टफ़ैक्ट बनाने की प्रोसेस की जांच करना
cd ${odp_fcp_github_repo}/python
bazel run //python/taskbuilder:task_builder_client -- --saved_model=${path_of_cloud_storage}/mnist_model/ --task_config=${path_of_cloud_storage}/mnist_cnn_task_config_build_artifact_only.pbtxt --build_artifact_only=true --task_builder_server=${task_builder_server_endpoint}
सैंपल मॉडल की पुष्टि, फ़्लेक्स ऑपरेशन की जांच और डीपी की जांच, दोनों के ज़रिए की जाती है. पुष्टि के दौरान बायपास करने के लिए, skip_flex_ops_check
और skip_dp_check
जोड़े जा सकते हैं. कुछ फ़्लेक्स ऑपरेशन मौजूद न होने की वजह से, इस मॉडल को ODP क्लाइंट के मौजूदा वर्शन में डिप्लॉय नहीं किया जा सकता.
cd ${odp_fcp_github_repo}/python
bazel run //python/taskbuilder:task_builder_client -- --saved_model=${path_of_cloud_storage}/mnist_model/ --task_config=${path_of_cloud_storage}/mnist_cnn_task_config_build_artifact_only.pbtxt --build_artifact_only=true --task_builder_server=${task_builder_server_endpoint} --skip_flex_ops_check=True --skip_dp_check=True
flex_ops_check: TensorFlow Lite में पहले से मौजूद ऑपरेटर लाइब्रेरी, सिर्फ़ कुछ ऑपरेटर के साथ काम करती है (TensorFlow Lite और TensorFlow ऑपरेटर के साथ काम करने की सुविधा). काम न करने वाले सभी tensorflow ऑपरेशन को फ़्लेक्स डेलिगेट (Android.bp) का इस्तेमाल करके इंस्टॉल करना ज़रूरी है. अगर किसी मॉडल में ऐसे ऑपरेशन शामिल हैं जो काम नहीं करते, तो उन्हें रजिस्टर करने के लिए हमसे संपर्क करें:
Cannot build the ClientOnlyPlan: Please contact Google to register these ops: {...}
टास्क बिल्डर को डीबग करने का सबसे अच्छा तरीका यह है कि उसे स्थानीय तौर पर शुरू किया जाए:
# Starts a server at localhost:5000 bazel run //python/taskbuilder:task_builder # Links to a server at localhost:5000 by removing task_builder_server flag bazel run //python/taskbuilder:task_builder_client -- --saved_model=${path_of_cloud_storage}/mnist_model/ --task_config=${path_of_cloud_storage}/mnist_cnn_task_config_build_artifact_only.pbtxt --build_artifact_only=true --skip_flex_ops_check=True --skip_dp_check=True
कॉन्फ़िगरेशन में बताए गए क्लाउड स्टोरेज में, आपको बनाए गए आर्टफ़ैक्ट दिखेंगे. यह कुछ ऐसा होना चाहिए जो GitHub रिपॉज़िटरी में दिए गए उदाहरण की तरह दिखना चाहिए.
पांचवां चरण. आर्टफ़ैक्ट बनाएं और एफ़सीपी सर्वर पर ट्रेनिंग और रेट के टास्क का एक नया जोड़ा बनाएं.
build_artifact_only
फ़्लैग हटाएं. इसके बाद, बने आर्टफ़ैक्ट, FCP सर्वर पर अपलोड हो जाएंगे. आपको यह देखना चाहिए कि ट्रेनिंग और आकलन के लिए, एक-दूसरे से जुड़े टास्क सही तरीके से बनाए गए हैं या नहीं
cd ${odp_fcp_github_repo}/python
bazel run //python/taskbuilder:task_builder_client -- --saved_model=${path_of_cloud_storage}/mnist_model/ --task_config=${path_of_cloud_storage}/mnist_cnn_task_config.pbtxt --task_builder_server=${task_builder_server_endpoint}
छठा चरण. एफ़सीपी क्लाइंट-साइड तैयार रखें
- ट्रेनिंग डेटा जनरेट करने के लिए, ODP का सार्वजनिक एपीआई
IsolatedWorker#onTrainingExamples
लागू करें. FederatedComputeScheduler#schedule
पर कॉल करें.- हमारे Android सोर्स रिपॉज़िटरी में कुछ उदाहरण देखें.
सातवां चरण. निगरानी
सर्वर मेट्रिक
हमारे GitHub रिपॉज़िटरी में, सेटअप करने के निर्देश देखें.
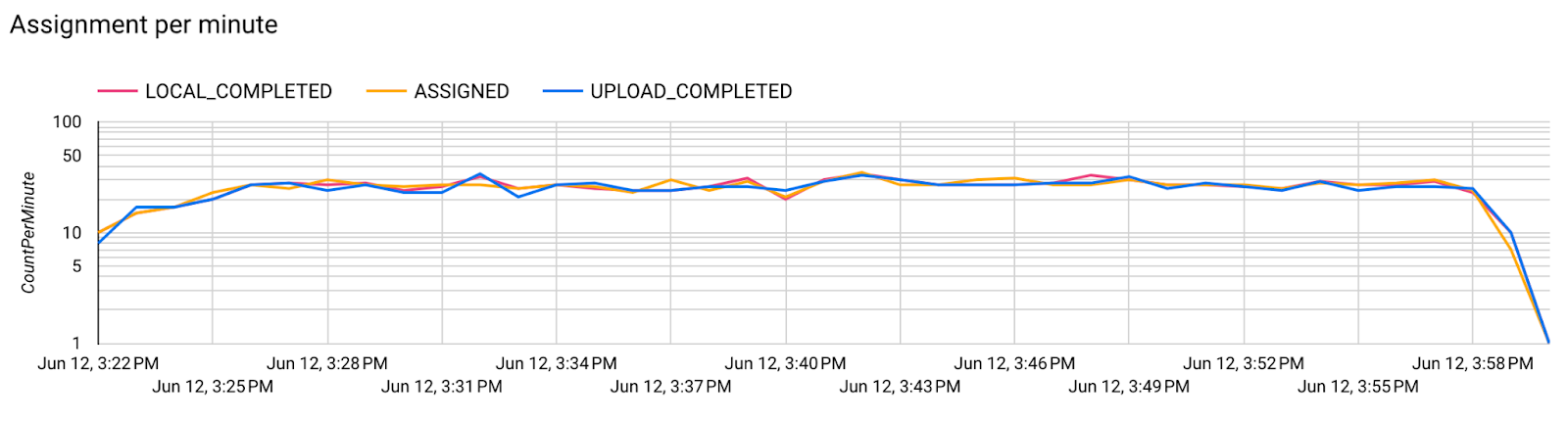
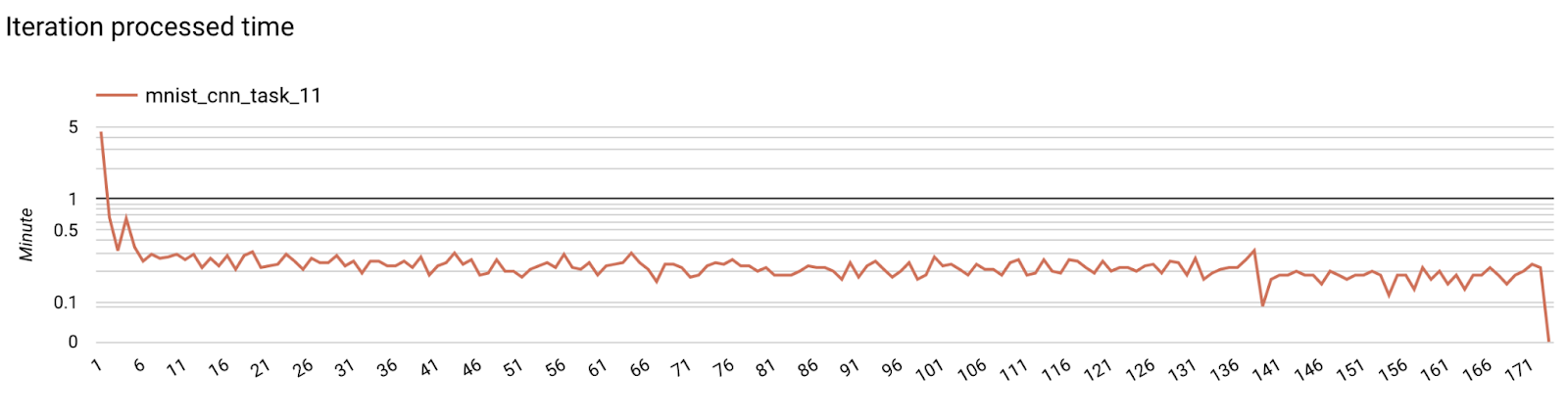
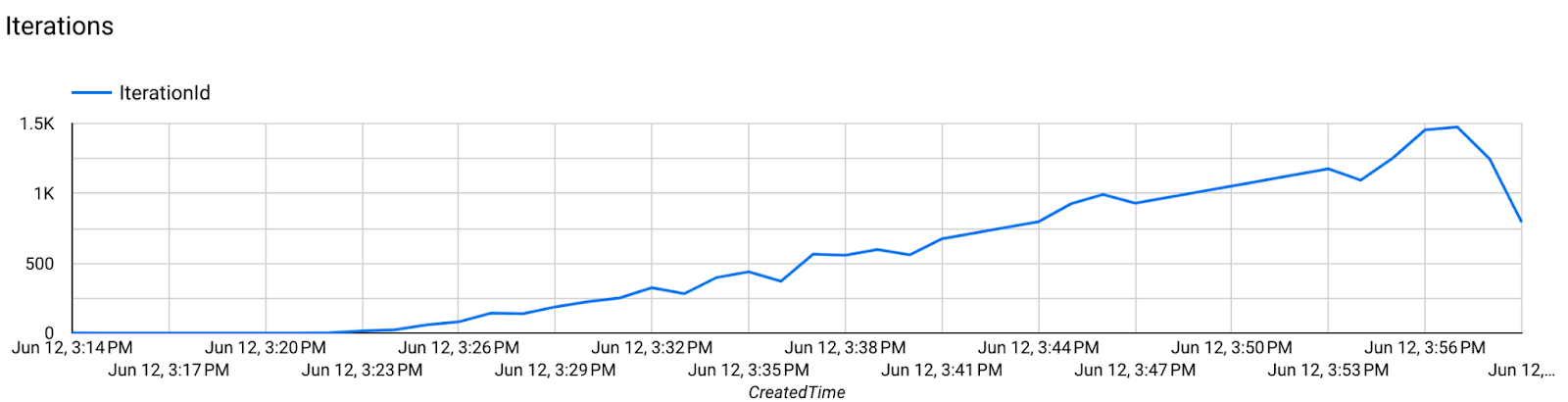
- मॉडल मेट्रिक
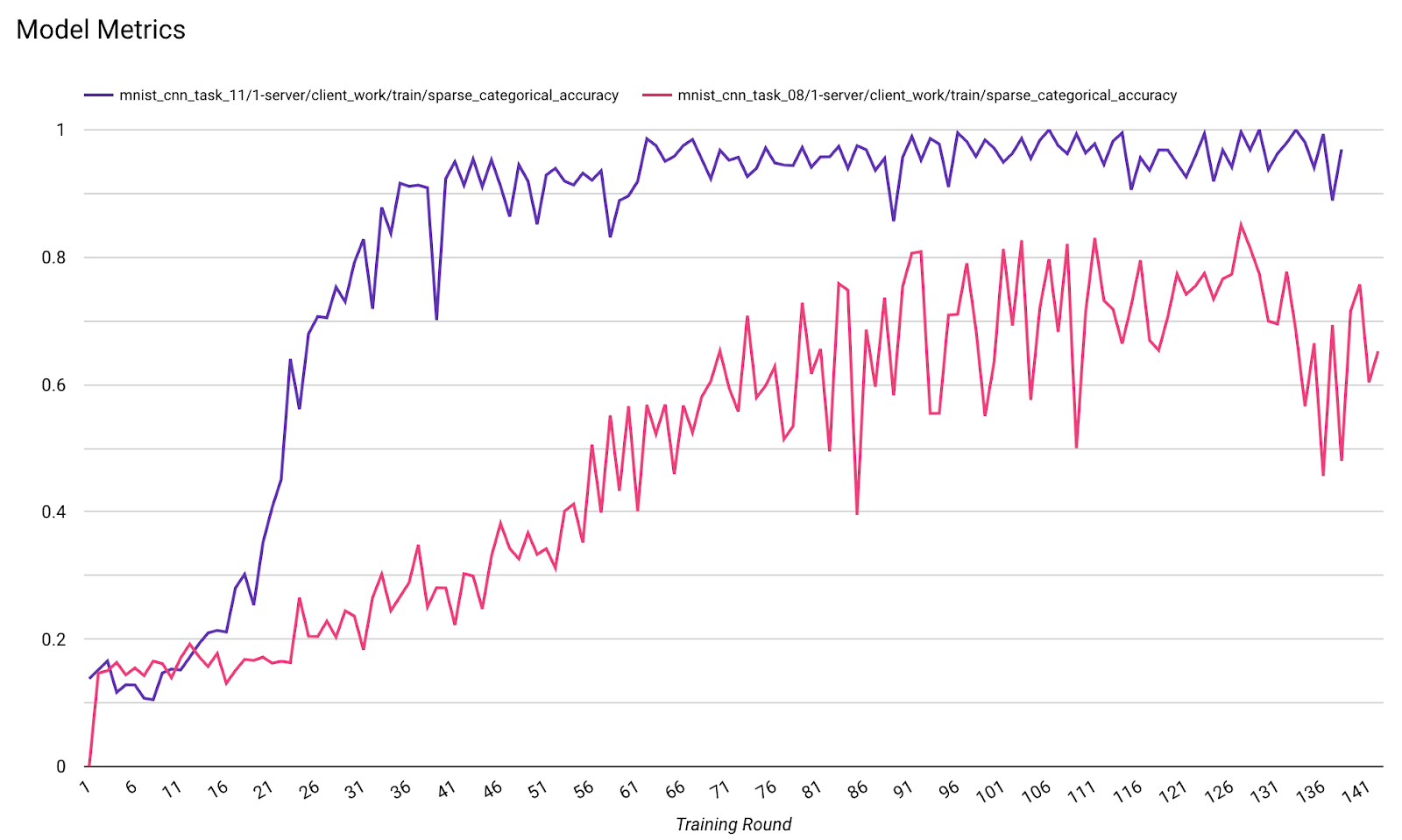
एक ही डायग्राम में अलग-अलग रनों की मेट्रिक की तुलना की जा सकती है. उदाहरण के लिए:
- बैंगनी रंग की लाइन
noise_multiplier
0.1 के साथ है - पिंक लाइन,
noise_multipiler
0.3 के साथ है