หน้านี้อธิบายวิธีทำงานกับ Federated Learning API จากการปรับเปลี่ยนในแบบของคุณในอุปกรณ์เพื่อฝึกโมเดลด้วยกระบวนการเรียนรู้โดยเฉลี่ยแบบสมาพันธ์และเสียงแบบเกาส์เชียนแบบคงที่
ก่อนเริ่มต้น
ก่อนเริ่มต้น ให้ทำตามขั้นตอนต่อไปนี้ในอุปกรณ์ทดสอบ
ตรวจสอบว่าได้ติดตั้งโมดูล OnDevicePersonalization แล้ว โมดูลนี้พร้อมให้ใช้งานผ่านการอัปเดตอัตโนมัติในเดือนเมษายน 2024
# List the modules installed on the device adb shell pm list packages --apex-only --show-versioncode
ตรวจสอบว่าโมดูลต่อไปนี้แสดงอยู่ในรายการโดยมีรหัสเวอร์ชัน 341717000 ขึ้นไป
package:com.google.android.ondevicepersonalization versionCode:341717000
หากโมดูลดังกล่าวไม่อยู่ในรายการ ให้ไปที่การตั้งค่า > ความปลอดภัยและความเป็นส่วนตัว > การอัปเดต > อัปเดตระบบ Google Play เพื่อให้แน่ใจว่าอุปกรณ์เป็นเวอร์ชันล่าสุด เลือกอัปเดตตามที่จำเป็น
เปิดใช้ฟีเจอร์ใหม่ทั้งหมดที่เกี่ยวข้องกับการเรียนรู้แบบรวมศูนย์
# Enable On-Device Personalization apk. adb shell device_config put on_device_personalization global_kill_switch false # Enable On-Device Personalization APIs. adb shell device_config put on_device_personalization enable_ondevicepersonalization_apis true # Enable On-Device Personalization overriding. adb shell device_config put on_device_personalization enable_personalization_status_override true adb shell device_config put on_device_personalization personalization_status_override_value true # Enable Federated Compute apk. adb shell device_config put on_device_personalization federated_compute_kill_switch false
สร้างงานการเรียนรู้แบบรวมศูนย์
ตัวเลขในแผนภาพที่มีการอธิบายอย่างละเอียดมากขึ้นใน 8 ขั้นตอนต่อไปนี้
กำหนดค่าเซิร์ฟเวอร์ Compute แบบรวมศูนย์
การเรียนรู้แบบรวมศูนย์คือ Map-Reduce ที่ทำงานบนเซิร์ฟเวอร์ประมวลผลแบบรวมศูนย์ (ตัวลด) และชุดไคลเอ็นต์ (ตัวแมป) เซิร์ฟเวอร์ Federated Compute จะดูแลข้อมูลเมตาและข้อมูลโมเดลที่ทำงานอยู่ของงานการเรียนรู้แบบรวมศูนย์แต่ละงาน สรุปคร่าวๆ มีดังนี้
- นักพัฒนาซอฟต์แวร์การเรียนรู้แบบสมาพันธ์สร้างงานใหม่และอัปโหลดทั้งข้อมูลเมตาที่เรียกใช้งานและข้อมูลโมเดลไปยังเซิร์ฟเวอร์
- เมื่อไคลเอ็นต์ Federated Compute เริ่มคำขอมอบหมายงานใหม่ไปยังเซิร์ฟเวอร์ เซิร์ฟเวอร์จะตรวจสอบสิทธิ์ของงานและส่งคืนข้อมูลงานที่มีสิทธิ์
- เมื่อลูกค้า Federated Compute ทำการประมวลผลในเครื่องเสร็จแล้ว ก็จะส่งผลลัพธ์การประมวลผลเหล่านี้ไปยังเซิร์ฟเวอร์ จากนั้นเซิร์ฟเวอร์จะรวบรวมและเพิ่มสัญญาณรบกวนให้กับผลลัพธ์การคำนวณเหล่านี้ และใช้ผลลัพธ์กับโมเดลสุดท้าย
ดูข้อมูลเพิ่มเติมเกี่ยวกับแนวคิดเหล่านี้ได้ที่
- การเรียนรู้แบบสมาพันธ์: แมชชีนเลิร์นนิงแบบทำงานร่วมกันที่ไม่มีข้อมูลการฝึกอบรมแบบรวมศูนย์
- ก้าวสู่การเรียนรู้แบบรวมศูนย์ในวงกว้าง: การออกแบบระบบ (SysML 2019)
ODP ใช้การเรียนรู้แบบรวมศูนย์เวอร์ชันที่ปรับปรุงแล้ว ซึ่งมีการใช้สัญญาณรบกวนที่ปรับแล้ว (แบบรวมศูนย์) กับข้อมูลที่รวบรวมก่อนนำไปใช้กับโมเดล ขนาดของสัญญาณรบกวนทำให้กลุ่มข้อมูลรวมรักษาความเป็นส่วนตัวที่แตกต่าง
ขั้นตอนที่ 1 สร้างเซิร์ฟเวอร์ Compute แบบรวมศูนย์
ทำตามวิธีการในโปรเจ็กต์ Federated Compute เพื่อตั้งค่า Federated Compute Server ของคุณเอง
ขั้นตอนที่ 2 เตรียม FunctionalModel ที่บันทึกไว้
เตรียมไฟล์ 'FunctionalModel' ที่บันทึกไว้ คุณสามารถใช้ 'functional_model_from_keras' เพื่อแปลง 'Model' เป็น 'FunctionalModel' และใช้ 'save_functional_model' เพื่อจัดรูปแบบ 'FunctionalModel' นี้เป็น 'SavedModel'
functional_model = tff.learning.models.functional_model_from_keras(keras_model=model)
tff.learning.models.save_functional_model(functional_model, saved_model_path)
ขั้นตอนที่ 3 สร้างการกำหนดค่า Federated Compute Server
เตรียม fcp_server_config.json
ซึ่งประกอบด้วยนโยบาย การตั้งค่าการเรียนรู้แบบรวมศูนย์ และการตั้งค่าความเป็นส่วนตัวแบบที่แตกต่างกัน ตัวอย่าง
# Identifies the set of client devices that will participate.
population_name: "my_new_population"
# Options you can choose:
# * TRAINING_ONLY: Only one training task will be generated under this
# population.
# * TRAINING_AND_EVAL: One training task and one evaluation task will be
# generated under this population.
# * EVAL_ONLY: Only one evaluation task will be generated under this
# population.
mode: TRAINING_AND_EVAL
policies {
# Policy for sampling on-device examples. It is checked every time a
# device attempts to start a new training.
min_separation_policy {
# The minimum number of rounds before the same client participated.
minimum_separation: 3
}
# Policy for releasing training results to developers. It is checked
# when uploading a new task to the Federated Compute Server.
model_release_policy {
# Server stops training when number of training rounds reaches this
# number.
num_max_training_rounds: 1000
}
}
# Federated learning setups. They are applied inside Task Builder.
federated_learning {
learning_process {
# Use FED_AVG to build federated learning process. Options you can
# choose:
# * FED_AVG: Federated Averaging algorithm
# (https://arxiv.org/abs/2003.00295)
# * FED_SDG: Federated SGD algorithm
# (https://arxiv.org/abs/1602.05629)
type: FED_AVG
# Optimizer used at client side training. Options you can choose:
# * ADAM
# * SGD
client_optimizer: SGD
# Learning rate used at client side training.
client_learning_rate: 0.01
# Optimizer used at server side training. Options you can choose:
# * ADAM
# * SGD
server_optimizer: ADAM
# Learning rate used at server side training.
sever_learning_rate: 1
runtime_config {
# Number of participating devices for each round of training.
report_goal: 2000
}
# List of metrics to be evaluated by the model during training and
# evaluation. Federated Compute Server provides a list of allowed
# metrics.
metrics {
name: "auc-roc"
}
metrics {
name: "binary_accuracy"
}
}
# Whether or not to generate a corresponding evaluation task under the same
# population. If this field isn't set, only one training task is
# generated under this population.
evaluation {
# The task id under the same population of the source training task that
# this evaluation task evaluates.
source_training_task_id: 1
# Decides how checkpoints from the training task are chosen for
# evaluation.
# * every_k_round: the evaluation task randomly picks one checkpoint
# from the past k rounds of training task checkpoints.
# * every_k_hour: the evaluation task randomly picks one checkpoint
# from the past k hours of training task checkpoints.
checkpoint_selector: "every_1_round"
# The traffic of this evaluation task in this population.
evaluation_traffic: 0.1
# Number of participating devices for each round of evaluation.
report_goal: 200
}
}
# Differential Privacy setups. They are applied inside the Task Builder.
differential_privacy {
# The DP aggregation algorithm you want to use. Options you can choose:
# * FIXED_GAUSSIAN: Federated Learning DP-SGD with fixed clipping norm
# described in "Learning Differentially Private Recurrent
# Language Models" (https://arxiv.org/abs/1710.06963).
# * ADAPTIVE_GAUSSIAN: Federated Learning DP-SGD with quantile-based clip
# norm estimation described in "Differentially Private
# Learning with Adaptive Clipping"
# (https://arxiv.org/abs/1905.03871).
# * TREE: DP-FTRL algorithm described in "Practical and Private (Deep)
# Learning without Sampling or Shuffling"
# (https://arxiv.org/abs/2103.00039).
# * ADADPTIVE_TREE: DP-FTRL with adaptive clipping norm descirbed in
# "Differentially Private Learning with Adaptive Clipping"
# (https://arxiv.org/abs/1905.03871).
type: FIXED_GAUSSIAN
# Noise multiplier for the Gaussian noise.
noise_multiplier: 0.1
# The value of the clipping norm.
clip_norm: 0.1
}
ขั้นตอนที่ 4 ส่งการกําหนดค่าไฟล์ ZIP ไปยังเซิร์ฟเวอร์ Federated Compute
ส่งไฟล์ ZIP และ fcp_server_config.json
ไปยังเซิร์ฟเวอร์ Federated Compute
task_builder_client --task_builder_server='http://{federated_compute_server_endpoint}' --saved_model='saved_model' --task_config='fcp_server_config.json'
ปลายทาง Federated Compute Server คือเซิร์ฟเวอร์ที่คุณตั้งค่าไว้ในขั้นตอนที่ 1
ไลบรารีโอเปอเรเตอร์ในตัวของ LiteRT รองรับโอเปอเรเตอร์ TensorFlow จํานวนจํากัดเท่านั้น (โอเปอเรเตอร์ TensorFlow บางรายการ) ชุดโอเปอเรเตอร์ที่รองรับอาจแตกต่างกันไปตามเวอร์ชันต่างๆ ของโมดูล OnDevicePersonalization กระบวนการยืนยันผู้ให้บริการจะดำเนินการภายในเครื่องมือสร้างงานระหว่างการสร้างงานเพื่อให้มั่นใจว่าเข้ากันได้
เวอร์ชันโมดูล OnDevicePersonalization ขั้นต่ำที่รองรับจะรวมอยู่ในข้อมูลเมตาของงาน ข้อมูลนี้ดูได้ในส่วนข้อความข้อมูลของเครื่องมือสร้างงาน
I1023 22:16:53.058027 139653371516736 task_builder_client.py:109] Success! Tasks are built, and artifacts are uploaded to the cloud. I1023 22:16:53.058399 139653371516736 task_builder_client.py:112] applied_algorithms { learning_algo: FED_AVG client_optimizer: SGD server_optimizer: SGD dp_aggregator: FIXED_GAUSSIAN } metric_results { accepted_metrics: "binary_accuracy, binary_crossentropy, recall, precision, auc-roc, auc-pr" } dp_hyperparameters { dp_delta: 0.000001 dp_epsilon: 6.4 noise_multiplier: 1.0 dp_clip_norm: 1.0 num_training_rounds: 10000 } I1023 22:16:53.058594 139653371516736 task_builder_client.py:113] training_task { min_client_version: "341912000" } eval_task { min_client_version: "341812000" }
เซิร์ฟเวอร์ Federated Compute จะมอบหมายงานนี้ให้กับอุปกรณ์ทั้งหมดที่มีโมดูล OnDevicePersonalization ซึ่งมีเวอร์ชันสูงกว่า 341812000
หากโมเดลของคุณมีการดำเนินการที่โมดูล OnDevicePersonalization ไม่รองรับ ระบบจะสร้างข้อความแสดงข้อผิดพลาดระหว่างการสร้างงาน
common.TaskBuilderException: Cannot build the ClientOnlyPlan: Please contact Google to register these ops: {'L2Loss': 'L2LossOp<CPUDevice, float>'} . Stop building remaining artifacts.
ดูรายการการดำเนินการแบบยืดหยุ่นที่รองรับโดยละเอียดได้ใน GitHub
สร้าง APK ของ Android Federated Compute
หากต้องการสร้าง APK ของ Federated Compute สำหรับ Android คุณต้องระบุปลายทาง URL ของเซิร์ฟเวอร์ Federated Compute ใน AndroidManifest.xml
ซึ่งไคลเอ็นต์ Federated Compute เชื่อมต่ออยู่
ขั้นตอนที่ 5 ระบุปลายทาง URL ของ Federated Compute Server
ระบุปลายทาง URL ของเซิร์ฟเวอร์ Federated Compute (ที่คุณตั้งค่าไว้ในขั้นตอนที่ 1) ใน AndroidManifest.xml
ซึ่งไคลเอ็นต์ Federated Compute เชื่อมต่ออยู่
<!-- Contents of AndroidManifest.xml -->
<manifest xmlns:android="http://schemas.android.com/apk/res/android"
package="com.example.odpsample" >
<application android:label="OdpSample">
<!-- XML resource that contains other ODP settings. -->
<property android:name="android.ondevicepersonalization.ON_DEVICE_PERSONALIZATION_CONFIG"
android:resource="@xml/OdpSettings"></property>
<!-- The service that ODP will bind to. -->
<service android:name="com.example.odpsample.SampleService"
android:exported="true" android:isolatedProcess="true" />
</application>
</manifest>
ไฟล์ทรัพยากร XML ที่ระบุในแท็ก <property>
จะต้องประกาศคลาสบริการในแท็ก <service>
ด้วย และระบุปลายทาง URL ของเซิร์ฟเวอร์ Compute แบบรวมศูนย์ที่ไคลเอ็นต์ Compute แบบรวมศูนย์จะเชื่อมต่อด้วย
<!-- Contents of res/xml/OdpSettings.xml -->
<on-device-personalization>
<!-- Name of the service subclass -->
<service name="com.example.odpsample.SampleService">
<!-- If you want to use federated compute feature to train a model,
specify this tag. -->
<federated-compute-settings url="https://fcpserver.com/" />
</service>
</on-device-personalization>
ขั้นตอนที่ 6 ใช้ IsolatedWorker#onTrainingExample
API
ใช้ IsolatedWorker#onTrainingExample
ของ API สาธารณะในการปรับเปลี่ยนอุปกรณ์ในแบบของคุณเพื่อสร้างข้อมูลการฝึก
โค้ดที่ทำงานใน IsolatedProcess
ไม่มีสิทธิ์เข้าถึงเครือข่าย ดิสก์ภายใน หรือบริการอื่นๆ ที่ทำงานในอุปกรณ์โดยตรง แต่จะใช้ API ต่อไปนี้ได้
- 'getRemoteData' - ข้อมูลคีย์-ค่าแบบคงที่ที่ดาวน์โหลดจากแบ็กเอนด์ระยะไกลที่นักพัฒนาแอปดำเนินการ หากมี
- 'getLocalData' - ข้อมูลคีย์-ค่าที่เปลี่ยนแปลงได้ซึ่งนักพัฒนาแอปคงอยู่ในเครื่อง หากมี
- "UserData" - ข้อมูลผู้ใช้ที่ได้จากแพลตฟอร์ม
- 'getLogReader' - แสดง DAO สำหรับตาราง "REQUESTS" และ "EVENTS"
ตัวอย่าง
@Override public void onTrainingExample(
@NonNull TrainingExampleInput input,
@NonNull Consumer<TrainingExampleOutput> consumer) {
// Check if the incoming training task is the task we want.
if (input.getPopulationName() == "my_new_population") {
TrainingExampleOutput result = new TrainingExampleOutput.Builder():
RequestLogRecord record = this.getLogReader().getRequestLogRecord(1);
int count = 1;
// Iterate logging event table.
for (ContentValues contentValues: record.rows()) {
Features features = Features.newBuilder()
// Retrieve carrier from user info.
.putFeature("carrier", buildFeature(mUserData.getCarrier()))
// Retrieve features from logging info.
.putFeature("int_feature_1",
buildFeature(contentValues.get("int_feature_1")
result.addTrainingExample(
Example.newBuilder()
.setFeatures(features).build().toByteArray())
.addResumptionToken(
String.format("token%d", count).getBytes()))
.build();
count++;
}
consumer.accept(result.build());
}
}
ขั้นตอนที่ 7 กำหนดเวลางานการฝึกอบรมที่เกิดซ้ำ
การปรับเปลี่ยนอุปกรณ์ในแบบของคุณมอบ FederatedComputeScheduler
ให้นักพัฒนาแอปกำหนดเวลาหรือยกเลิกงานการประมวลผลแบบรวมศูนย์ คุณสามารถเรียกใช้ผ่าน IsolatedWorker
ได้หลายวิธี ไม่ว่าจะเป็นตามกำหนดเวลาหรือเมื่อการดาวน์โหลดแบบไม่พร้อมกันเสร็จสมบูรณ์ ตัวอย่างของทั้ง 2 รูปแบบมีดังนี้
ตัวเลือกตามตารางเวลา โทรหา
FederatedComputeScheduler#schedule
ในIsolatedWorker#onExecute
@Override public void onExecute( @NonNull ExecuteInput input, @NonNull Consumer<ExecuteOutput> consumer ) { if (input != null && input.getAppParams() != null && input.getAppParams().getString("schedule_training") != null) { if (input.getAppParams().getString("schedule_training").isEmpty()) { consumer.accept(null); return; } TrainingInterval interval = new TrainingInterval.Builder() .setMinimumInterval(Duration.ofSeconds(10)) .setSchedulingMode(2) .build(); FederatedComputeScheduler.Params params = new FederatedComputeScheduler .Params(interval); FederatedComputeInput fcInput = new FederatedComputeInput.Builder() .setPopulationName( input.getAppParams().getString("schedule_training")).build(); mFCScheduler.schedule(params, fcInput); ExecuteOutput result = new ExecuteOutput.Builder().build(); consumer.accept(result); } }
ตัวเลือกการดาวน์โหลดเสร็จสมบูรณ์ เรียกใช้
FederatedComputeScheduler#schedule
ในIsolatedWorker#onDownloadCompleted
หากการจัดตารางเวลางานการฝึกขึ้นอยู่กับข้อมูลหรือกระบวนการแบบไม่พร้อมกัน
การตรวจสอบความถูกต้อง
ขั้นตอนต่อไปนี้อธิบายวิธีตรวจสอบว่างานการเรียนรู้แบบรวมศูนย์ทํางานได้อย่างถูกต้องหรือไม่
ขั้นตอนที่ 8 ตรวจสอบว่างานการเรียนรู้แบบรวมศูนย์ทํางานได้อย่างถูกต้อง
ระบบจะสร้างจุดตรวจสอบโมเดลใหม่และไฟล์เมตริกใหม่ในการรวมฝั่งเซิร์ฟเวอร์แต่ละรอบ
เมตริกอยู่ในไฟล์คู่คีย์-ค่ารูปแบบ JSON ไฟล์จะสร้างขึ้นจากรายการ Metrics
ที่คุณกำหนดไว้ในขั้นตอนที่ 3 ตัวอย่างไฟล์ JSON ของเมตริกตัวแทนมีดังนี้
{"server/client_work/train/binary_accuracy":0.5384615659713745, "server/client_work/train/binary_crossentropy":0.694046676158905, "server/client_work/train/recall":0.20000000298023224, "server/client_work/train/precision":0.3333333432674408, "server/client_work/train/auc-roc":0.3500000238418579, "server/client_work/train/auc-pr":0.44386863708496094, "server/finalizer/update_non_finite":0.0}
คุณสามารถใช้สคริปต์ที่คล้ายกับสคริปต์ต่อไปนี้เพื่อรับเมตริกของโมเดลและตรวจสอบประสิทธิภาพการฝึก
import collections
import json
import matplotlib.pyplot as plt
from google.cloud import storage
# The population_name you set in fcp_server_config.json in Step 3.
POPULATION_NAME = 'my_new_population'
# The Google Cloud storage you set in Step 1.
GCS_BUCKET_NAME = 'fcp-gcs'
NUM_TRAINING_ROUND = 1000
storage_client = storage.Client()
bucket = storage_client.bucket(GCS_BUCKET_NAME)
metrics = collections.defaultdict(list)
for i in range(NUM_TRAINING_ROUND):
blob = bucket.blob('{}/{}/1/{}/s/0/metrics'.format(GCS_BUCKET_NAME, POPULATION_NAME, i+1))
with blob.open("r") as f:
metric = json.loads(f.read())
for metric_name in metric.keys():
metrics[metric_name].append(metric[metric_name])
for metric_name in metrics:
print(metric_name)
plt.plot(metrics[metric_name])
plt.show()
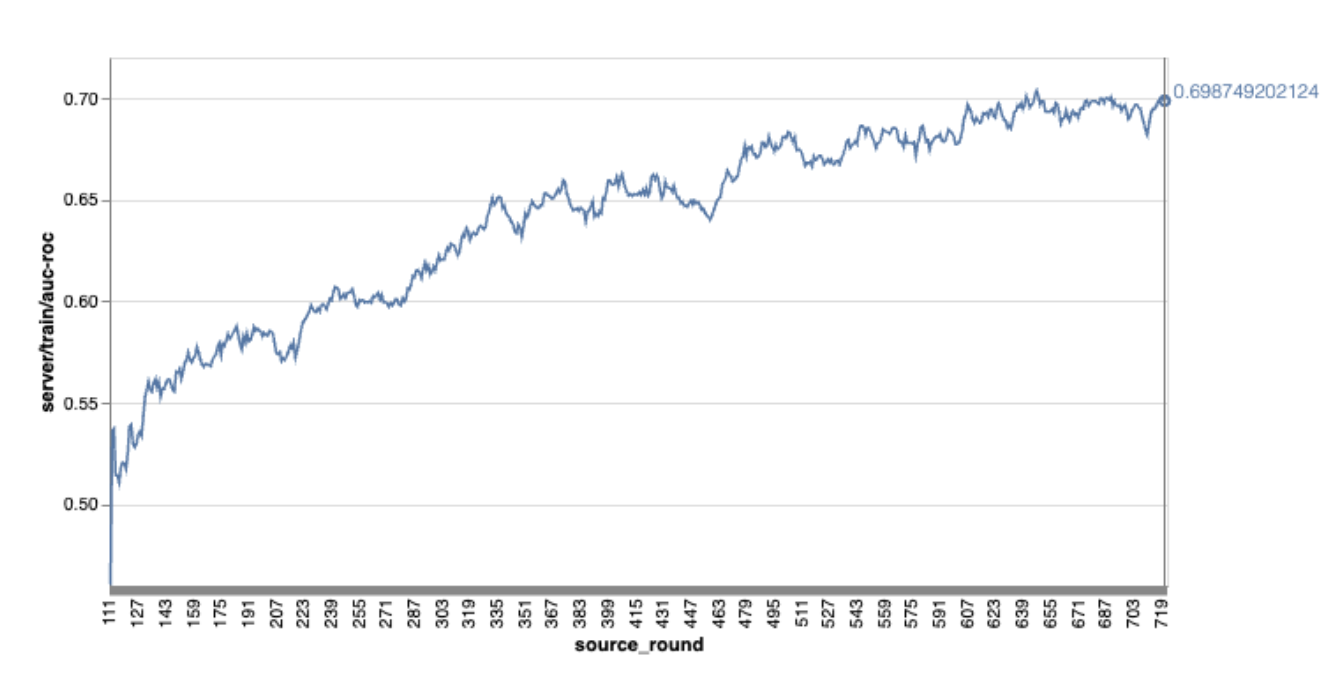
โปรดทราบว่าในกราฟตัวอย่างก่อนหน้านี้
- แกน X คือจํานวนรอบการฝึก
- แกน y คือค่าของ auc-roc ของแต่ละรอบ
การฝึกโมเดลการจัดประเภทอิมเมจในการปรับเปลี่ยนในแบบของคุณในอุปกรณ์
ในบทแนะนำนี้ เราจะใช้ชุดข้อมูล EMNIST เพื่อสาธิตวิธีเรียกใช้งานการเรียนรู้แบบรวมศูนย์ใน ODP
ขั้นตอนที่ 1 สร้าง tff.learning.models.FunctionalModel
def get_image_classification_input_spec():
return (
tf.TensorSpec([None, 28, 28, 1], tf.float32),
tf.TensorSpec([None, 1], tf.int64),
)
def create_and_save_image_classification_functional_model(
model_path: str,
) -> None:
keras_model = emnist_models.create_original_fedavg_cnn_model(
only_digits=True
)
functional_model = tff.learning.models.functional_model_from_keras(
keras_model=keras_model,
input_spec=get_image_classification_input_spec(),
loss_fn=tf.keras.losses.SparseCategoricalCrossentropy(),
)
tff.learning.models.save_functional_model(functional_model, model_path)
- คุณดูรายละเอียดโมเดล emnist keras ได้ใน emnist_models
- TfLite ยังไม่รองรับ tf.sparse.SparseTensor หรือ tf.RaggedTensor พยายามใช้ tf.Tensor ให้มากที่สุดเท่าที่จะเป็นไปได้เมื่อสร้างโมเดล
- เครื่องมือสร้างงาน ODP จะเขียนทับเมตริกทั้งหมดเมื่อสร้างกระบวนการเรียนรู้ คุณไม่จำเป็นต้องระบุเมตริกใดๆ เราจะกล่าวถึงหัวข้อนั้นเพิ่มเติมในขั้นตอนที่ 2 สร้างการกำหนดค่าเครื่องมือสร้างงาน
อินพุตโมเดล 2 ประเภทที่รองรับ ได้แก่
ประเภทที่ 1 Tuple(features_tensor, label_tensor)
- เมื่อสร้างโมเดล Input_spec จะมีลักษณะดังนี้
def get_input_spec(): return ( tf.TensorSpec([None, 28, 28, 1], tf.float32), tf.TensorSpec([None, 1], tf.int64), )
- จับคู่รายการก่อนหน้ากับการใช้งาน API สาธารณะของ ODP IsolatedWorker#onTrainingExamples ต่อไปนี้เพื่อสร้างข้อมูลการฝึกในอุปกรณ์
return tf.train.Example( features=tf.train.Features( feature={ 'x': tf.train.Feature( float_list=tf.train.FloatList(value=[1.0] * 784) ), 'y': tf.train.Feature( int64_list=tf.train.Int64List( value=[1] ) ), } ) ).SerializeToString()
ประเภท 2
Tuple(Dict[feature_name, feature_tensor], label_tensor)
- เมื่อสร้างโมเดล Input_spec จะมีลักษณะดังนี้
def get_input_spec() -> ( Tuple[collections.OrderedDict[str, tf.TensorSpec], tf.TensorSpec] ): return ( collections.OrderedDict( [('feature-1', tf.TensorSpec([None, 1], tf.float32)), ('feature-2', tf.TensorSpec([None, 1], tf.float32))] ), tf.TensorSpec([None, 1], tf.int64), )
- จับคู่รายการก่อนหน้ากับการใช้งาน API สาธารณะของ ODP IsolatedWorker#onTrainingExamples ต่อไปนี้เพื่อสร้างข้อมูลการฝึก
return tf.train.Example( features=tf.train.Features( feature={ 'feature-1': tf.train.Feature( float_list=tf.train.FloatList(value=[1.0]) ), 'feature-2': tf.train.Feature( float_list=tf.train.FloatList(value=[2.0]) ), 'my_label': tf.train.Feature( int64_list=tf.train.Int64List( value=[1] ) ), } ) ).SerializeToString()
- อย่าลืมลงทะเบียน label_name ในการกำหนดค่าเครื่องมือสร้างงาน
mode: TRAINING_AND_EVAL # Task execution mode population_name: "my_example_model" label_name: "my_label"
ODP จะจัดการ DP โดยอัตโนมัติเมื่อสร้างกระบวนการเรียนรู้ คุณจึงไม่จำเป็นต้องเพิ่มสัญญาณรบกวนใดๆ เมื่อสร้างโมเดลการทํางาน
เอาต์พุตของโมเดลการทำงานที่บันทึกไว้นี้ควรมีลักษณะเหมือนตัวอย่างในที่เก็บ GitHub ของเรา
ขั้นตอนที่ 2 สร้างการกำหนดค่าเครื่องมือสร้างงาน
คุณดูตัวอย่างการกำหนดค่าเครื่องมือสร้างงานได้ที่ที่เก็บ GitHub ของเรา
เมตริกการฝึกอบรมและการประเมิน
เนื่องจากเมตริกอาจรั่วไหลข้อมูลผู้ใช้ ตัวสร้างงานจะมีรายการเมตริกที่กระบวนการเรียนรู้สามารถสร้างและเผยแพร่ได้ คุณดูรายการทั้งหมดได้ในที่เก็บ GitHub ของเรา
ต่อไปนี้คือตัวอย่างรายการเมตริกเมื่อสร้างการกำหนดค่าเครื่องมือสร้างงานใหม่
federated_learning { learning_process { metrics { name: "binary_accuracy" } metrics { name: "binary_crossentropy" } metrics { name: "recall" } metrics { name: "precision" } metrics { name: "auc-roc" } metrics { name: "auc-pr" } } }
หากเมตริกที่คุณสนใจไม่อยู่ในรายการปัจจุบัน โปรดติดต่อเรา
การกำหนดค่า DP
มีการกำหนดค่าที่เกี่ยวข้องกับ DP บางรายการซึ่งต้องระบุข้อมูลต่อไปนี้
policies { min_separation_policy { minimum_separation: 1 } model_release_policy { num_max_training_rounds: 1000 dp_target_epsilon: 10 dp_delta: 0.000001 } } differential_privacy { type: FIXED_GAUSSIAN clip_norm: 0.1 noise_multiplier: 0.1 }
- มี
dp_target_epsilon
หรือnoise_mulitipiler
อยู่เพื่อให้ผ่านการตรวจสอบความถูกต้อง: (noise_to_epsilon
epislon_to_noise
) - คุณดูการตั้งค่าเริ่มต้นเหล่านี้ได้ที่ที่เก็บ GitHub ของเรา
- มี
ขั้นตอนที่ 3 อัปโหลดการกำหนดค่าโมเดลและเครื่องมือสร้างงานที่บันทึกไว้ไปยังพื้นที่เก็บข้อมูลระบบคลาวด์ของนักพัฒนาซอฟต์แวร์รายใดก็ได้
อย่าลืมอัปเดตช่อง artifact_building เมื่ออัปโหลดการกำหนดค่าเครื่องมือสร้างงาน
ขั้นตอนที่ 4 (ไม่บังคับ) ทดสอบการสร้างอาร์ติแฟกต์โดยไม่ต้องสร้างงานใหม่
cd ${odp_fcp_github_repo}/python
bazel run //python/taskbuilder:task_builder_client -- --saved_model=${path_of_cloud_storage}/mnist_model/ --task_config=${path_of_cloud_storage}/mnist_cnn_task_config_build_artifact_only.pbtxt --build_artifact_only=true --task_builder_server=${task_builder_server_endpoint}
โมเดลตัวอย่างได้รับการตรวจสอบผ่านทั้งการตรวจสอบการดำเนินการแบบยืดหยุ่นและการตรวจสอบ dp คุณสามารถเพิ่ม skip_flex_ops_check
และ skip_dp_check
เพื่อข้ามการตรวจสอบได้ (โมเดลนี้ไม่สามารถนำไปใช้งานกับไคลเอ็นต์ ODP เวอร์ชันปัจจุบันได้เนื่องจากไม่มีการดำเนินการแบบยืดหยุ่นบางรายการ)
cd ${odp_fcp_github_repo}/python
bazel run //python/taskbuilder:task_builder_client -- --saved_model=${path_of_cloud_storage}/mnist_model/ --task_config=${path_of_cloud_storage}/mnist_cnn_task_config_build_artifact_only.pbtxt --build_artifact_only=true --task_builder_server=${task_builder_server_endpoint} --skip_flex_ops_check=True --skip_dp_check=True
flex_ops_check: ไลบรารีโอเปอเรเตอร์ TensorFlow Lite ในตัวรองรับโอเปอเรเตอร์ TensorFlow จำนวนจำกัดเท่านั้น (ความเข้ากันได้ของโอเปอเรเตอร์ TensorFlow Lite และ TensorFlow) คุณต้องติดตั้งการดำเนินการ tensorflow ทั้งหมดที่ใช้ร่วมกันไม่ได้โดยใช้ตัวรับมอบสิทธิ์ Flex (Android.bp) หากโมเดลมีการดำเนินการที่ไม่รองรับ โปรดติดต่อเราเพื่อลงทะเบียน
Cannot build the ClientOnlyPlan: Please contact Google to register these ops: {...}
วิธีที่ดีที่สุดในการแก้ไขข้อบกพร่องของเครื่องมือสร้างงานคือการเริ่มต้น 1 อย่างในเครื่อง
# Starts a server at localhost:5000 bazel run //python/taskbuilder:task_builder # Links to a server at localhost:5000 by removing task_builder_server flag bazel run //python/taskbuilder:task_builder_client -- --saved_model=${path_of_cloud_storage}/mnist_model/ --task_config=${path_of_cloud_storage}/mnist_cnn_task_config_build_artifact_only.pbtxt --build_artifact_only=true --skip_flex_ops_check=True --skip_dp_check=True
คุณดูอาร์ติแฟกต์ที่ได้ได้ที่ที่เก็บข้อมูลระบบคลาวด์ที่ระบุในการกําหนดค่า ซึ่งควรเป็นรูปแบบที่คล้ายกับตัวอย่างในที่เก็บ GitHub
ขั้นตอนที่ 5 สร้างอาร์ติแฟกต์และสร้างงานการฝึกและการประเมินคู่ใหม่บนเซิร์ฟเวอร์ FCP
นำ Flag build_artifact_only
ออก ระบบจะอัปโหลดอาร์ติแฟกต์ที่สร้างขึ้นไปยังเซิร์ฟเวอร์ FCP คุณควรตรวจสอบว่ามีการสร้างงานการฝึกและการประเมินคู่สำเร็จแล้ว
cd ${odp_fcp_github_repo}/python
bazel run //python/taskbuilder:task_builder_client -- --saved_model=${path_of_cloud_storage}/mnist_model/ --task_config=${path_of_cloud_storage}/mnist_cnn_task_config.pbtxt --task_builder_server=${task_builder_server_endpoint}
ขั้นตอนที่ 6 เตรียมฝั่งไคลเอ็นต์ FCP ให้พร้อม
- ใช้ ODP Public API
IsolatedWorker#onTrainingExamples
เพื่อสร้างข้อมูลการฝึก - โทร
FederatedComputeScheduler#schedule
- ดูตัวอย่างบางส่วนในที่เก็บซอร์สโค้ด Android
ขั้นตอนที่ 7 การตรวจสอบ
เมตริกเซิร์ฟเวอร์
ดูวิธีการตั้งค่าในที่เก็บ GitHub ของเรา
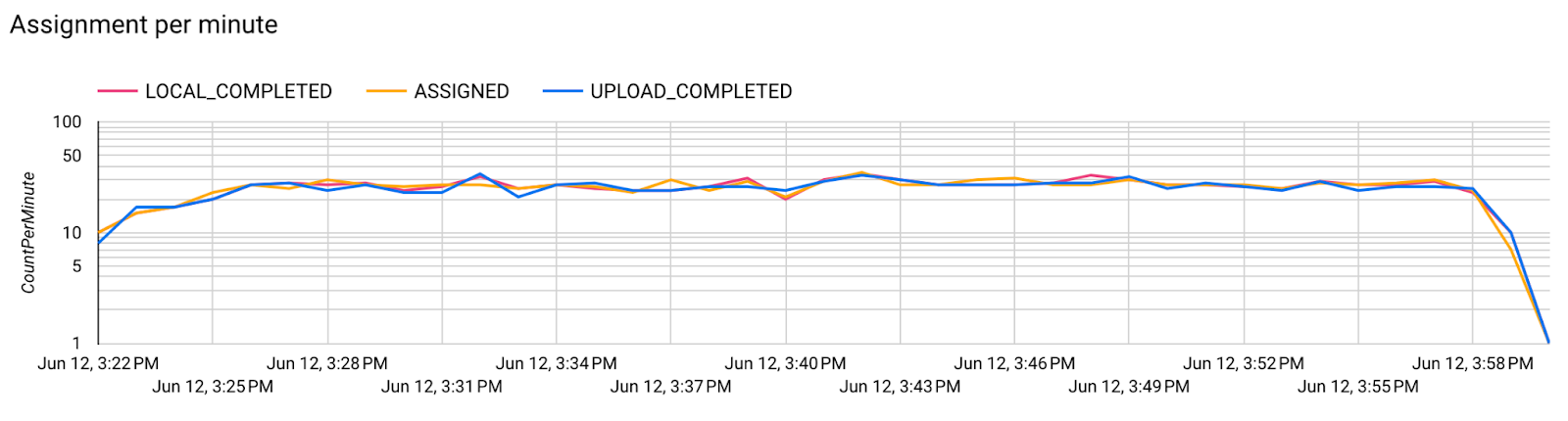
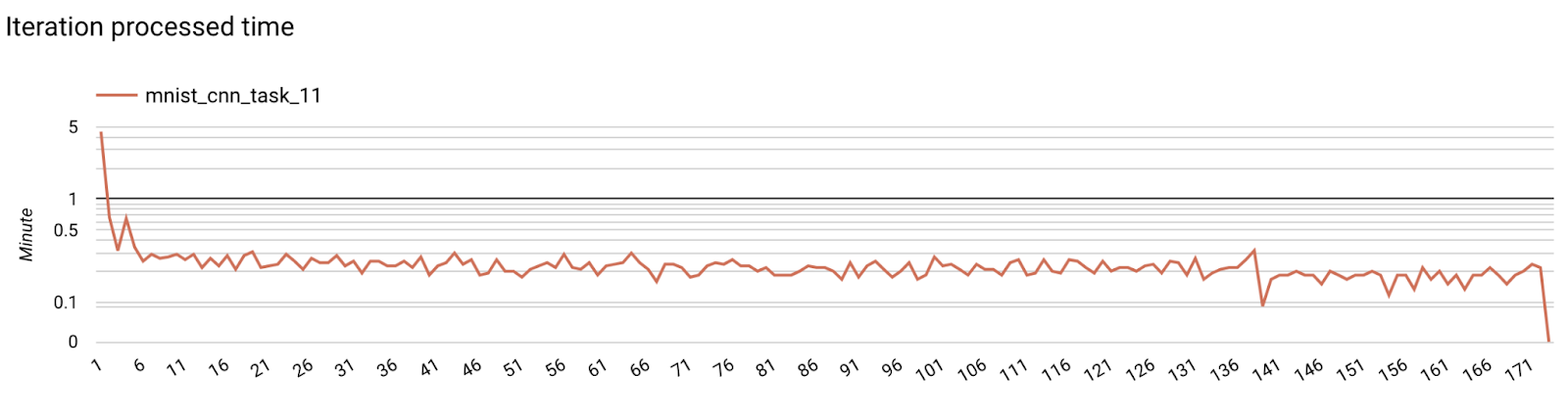
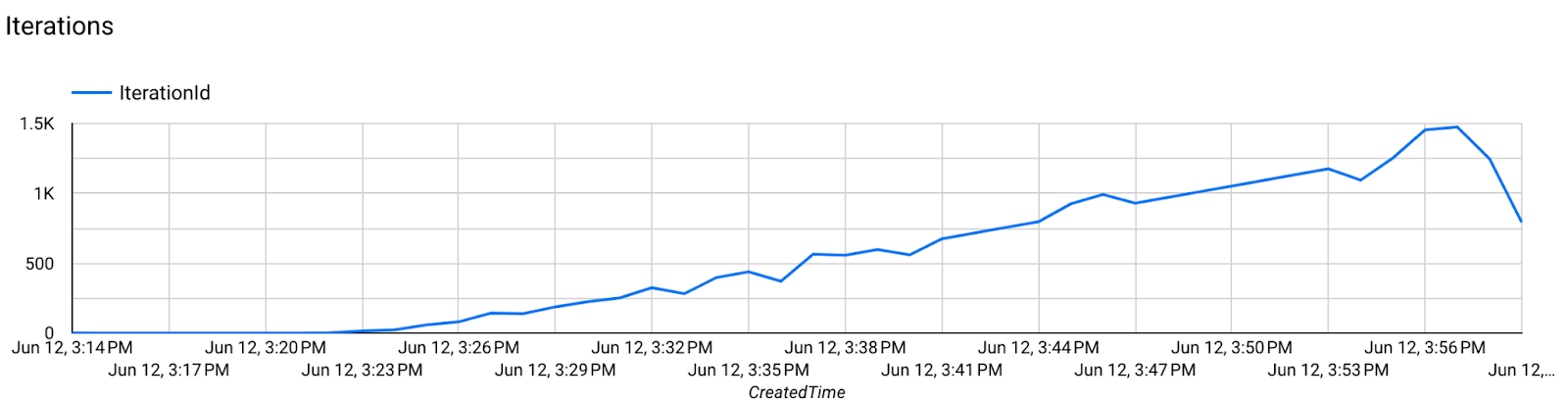
- เมตริกรูปแบบ
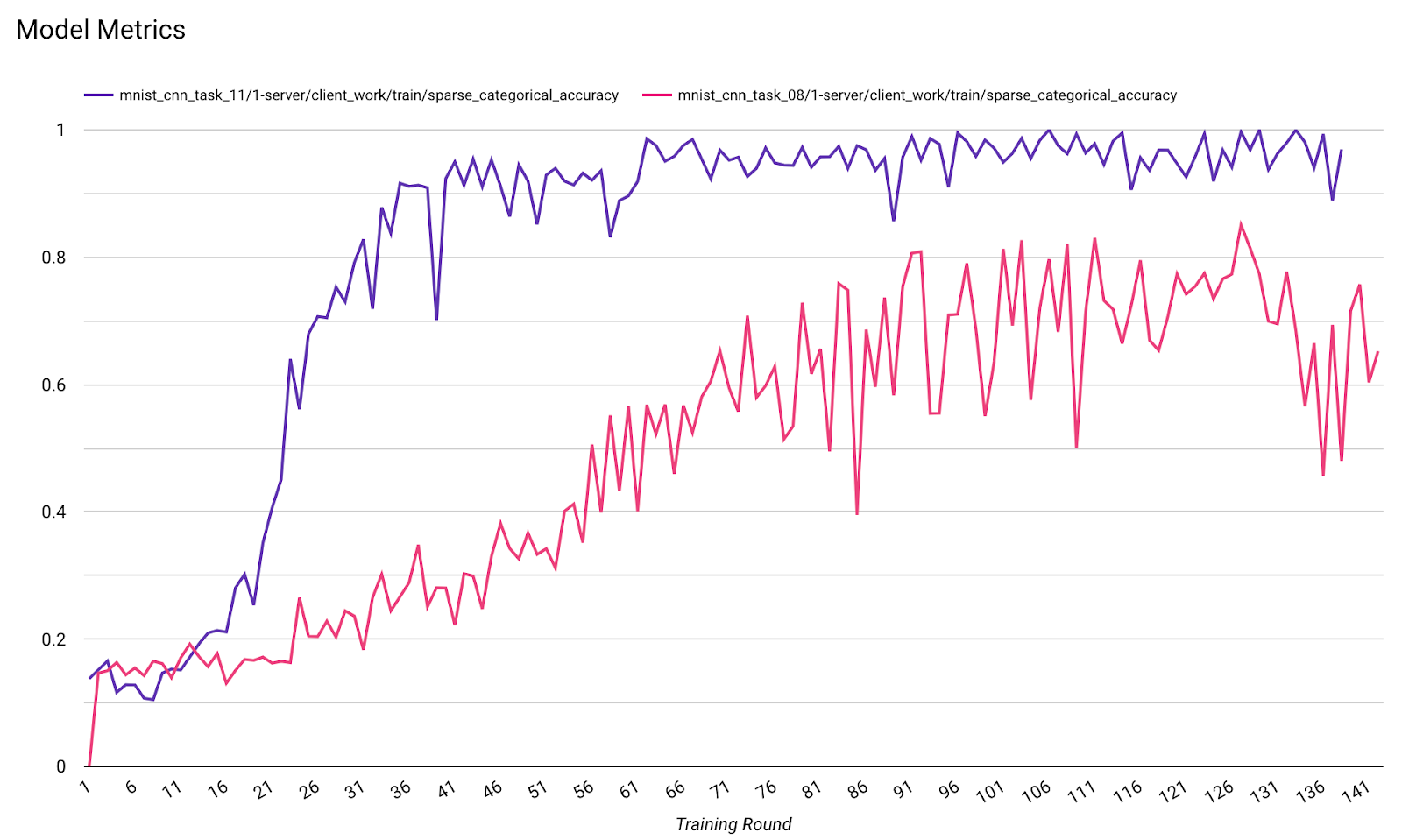
คุณสามารถเปรียบเทียบเมตริกจากการเรียกใช้ที่แตกต่างกันในแผนภูมิเดียวได้ เช่น
- เส้นสีม่วงมี
noise_multiplier
0.1 - เส้นสีชมพูคือ
noise_multipiler
0.3