隨機森林
這是牛津。
圖 19. 牛。
1906 年,英格蘭舉辦了重量級評選。
787 位參與者猜測了牛隻的體重。個別字詞的錯誤中位數為 37 磅 (錯誤為 3.1%)。但是,字詞的整體中位數與牛津的實際權重 (1198 磅) 相差 9 磅,誤差範圍只有 0.7%。
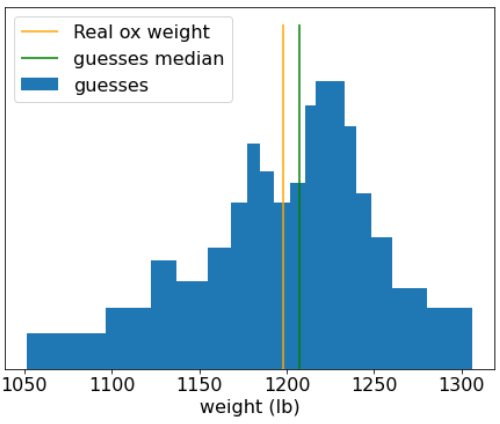
圖 20. 個別體重猜測的直方圖。
這個紀元展現了群眾的智慧:在特定情況下,集體意見有助於提高判斷力。
從數學上來說,模型的智慧會以中央限制定理進行模擬:理論上,值與這個值的 N 雜項預估值的平均值相差為 0,這個值為 1/N。不過,如果變數不是獨立變數,差異就會較大。
在機器學習中,集合是一組模型的預測平均值 (或某種方式匯總)。如果整組模型的大小差異很大,且不會造成個別不佳,則群集品質通常優於個別個別模型的品質。設定集需要的訓練和推論時間比單一模型來得長。畢竟,您必須針對多個模型 (而非單一模型) 執行訓練和推論。
相反地,為了讓整體作業發揮最佳效能,個別模型應獨立運作。以 10 個完全相同的模型 (也就是完全獨立) 組成的組圖來說,這個組合不會比個別模型更好。反之,如果強制獨立模型,可能會導致模型變得惡化。想有效進行分析,就必須在模型獨立性與子模型品質之間取得平衡。
除非另有註明,否則本頁面中的內容是採用創用 CC 姓名標示 4.0 授權,程式碼範例則為阿帕契 2.0 授權。詳情請參閱《Google Developers 網站政策》。Java 是 Oracle 和/或其關聯企業的註冊商標。
上次更新時間:2022-09-26 (世界標準時間)。
[null,null,["上次更新時間:2022-09-26 (世界標準時間)。"],[[["The \"wisdom of the crowd\" suggests that collective opinions can provide surprisingly accurate judgments, as demonstrated by a 1906 ox weight-guessing competition where the collective guess was remarkably close to the true weight."],["This phenomenon can be explained by the Central Limit Theorem, which states that the average of multiple independent estimates tends to converge towards the true value."],["In machine learning, ensembles leverage this principle by combining predictions from multiple models, improving overall accuracy when individual models are sufficiently diverse and reasonably accurate."],["While ensembles require more computational resources, their enhanced predictive performance often outweighs the added cost, especially when individual models are carefully selected and combined."],["Achieving optimal ensemble performance involves striking a balance between ensuring model independence to avoid redundant predictions and maintaining the individual quality of sub-models for overall accuracy."]]],[]]