随机森林
这是牛的声音。
图 19. 一头牛。
1906 年,英国进行了体重评选。787 名参与者猜测了一头牛的体重。各项猜测的中位数误差为 37 磅(误差为 3.1%)。不过,这些猜测的整体中位数只与牛的真实体重相距 9 磅(1198 磅),误差仅为 0.7%。
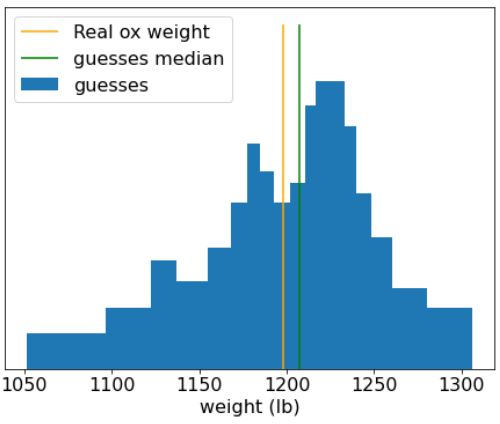
图 20. 单个体重猜测的直方图。
这则趣事揭示了群体的智慧:在某些情况下,集体意见提供了很好的判断力。
从数学上来讲,可以根据中心极限定理对群体的智慧进行建模:通俗地说,一个值与 N 个噪声估算值的平均值之间的平方误差往往为 1/N 的倍数。但是,如果变量不是独立的,方差就更大。
在机器学习中,集成是模型的预测结果的平均值(或以某种方式进行汇总)。如果集成模型足够不同,但又不过度糟糕,则集成学习的质量通常优于每个单独的模型的质量。与单个模型相比,集成需要更多的训练和推断时间。毕竟,您必须对多个模型(而不是单个模型)执行训练和推断。
通俗来说,为了在集成中发挥最佳效果,各个模型应该是独立的。举例来说,由 10 个完全相同的模型(即完全不独立)组成的集成学习不可能优于单个模型。另一方面,强制模型成为独立模型可能会使它们变得更糟糕。有效集成需要在模型独立性及其子模型的质量之间取得平衡。
如未另行说明,那么本页面中的内容已根据知识共享署名 4.0 许可获得了许可,并且代码示例已根据 Apache 2.0 许可获得了许可。有关详情,请参阅 Google 开发者网站政策。Java 是 Oracle 和/或其关联公司的注册商标。
最后更新时间 (UTC):2022-09-26。
[null,null,["最后更新时间 (UTC):2022-09-26。"],[[["The \"wisdom of the crowd\" suggests that collective opinions can provide surprisingly accurate judgments, as demonstrated by a 1906 ox weight-guessing competition where the collective guess was remarkably close to the true weight."],["This phenomenon can be explained by the Central Limit Theorem, which states that the average of multiple independent estimates tends to converge towards the true value."],["In machine learning, ensembles leverage this principle by combining predictions from multiple models, improving overall accuracy when individual models are sufficiently diverse and reasonably accurate."],["While ensembles require more computational resources, their enhanced predictive performance often outweighs the added cost, especially when individual models are carefully selected and combined."],["Achieving optimal ensemble performance involves striking a balance between ensuring model independence to avoid redundant predictions and maintaining the individual quality of sub-models for overall accuracy."]]],[]]